Home>European Law and Algorithmic Bias
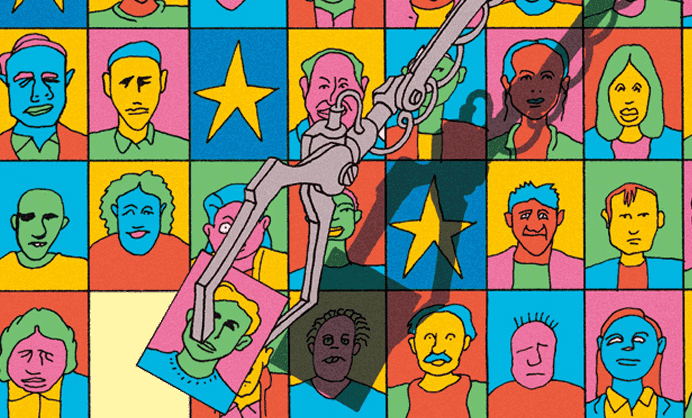
12.02.2025
European Law and Algorithmic Bias
The big data that platforms use to produce rankings, forecasts, and recommendations are anything but neutral. On the contrary, the platforms are major sources of discrimination of all kinds.
Although it has recently introduced a pioneering strategy for oversight in this area, the European regulator still has a long way to go. In particular, it is important to invest in understanding these systems so that we are able to devise effective preventive and corrective measures for both designers and users.
Read this interview with Raphaële Xenidis, Assistant Professor in European Law at Sciences Po Law School and Honorary Fellow at the University of Edinburgh School of Law. This article was originally published in the second issue of Understanding Our Times, Sciences Po Magazine.
flip through the full magazine
Your work includes an analysis of algorithmic discrimination and of challenges in combatting it. What do you mean by this?
Algorithmic discrimination stems from a process that may seem simple at first glance, but that raises some thorny questions. Algorithms draw on vast quantities of data (so-called big data), from which they make recommendations, predictions, rankings and risk assessments, or provide answers to questions they are asked, among other things.
But data is obviously not neutral; it reflects existing discrimination and inequalities. Let’s take the case of hiring for a role in a traditionally male-dominated profession, such as information technology (IT). Analysis of existing data (from previous hiring, for example) would bring forth male applicants and might lead an algorithm trained on this data to favour male applicants in the future. Eliminating this bias is not impossible, but the process uncovers other biases, since the over-representation of men in IT results from their over-representation in this discipline in higher education, and it is difficult to disregard qualifications when hiring.
The cases are legion: by using statistical data and profiling based on gender, finances, addresses, and user health and age, some algorithms might block users’ access to a given good or service, or offer them worse conditions without any examination of their actual characteristics.
A decision not to use certain discriminating parameters generally requires the use of other parameters that appear neutral but are, in fact, strongly correlated with sensitive data. For example, even if a salary criterion is jettisoned to avoid socioeconomic discrimination, an address could provide the algorithm with indications of an individual’s social class. This phenomenon, known as redundant encoding, can create discrimination by proxy, that is, arising from data that is a priori non-discriminatory but that actually encodes certain inequalities.
Furthermore, bias affects not only the data, but also every stage in the deployment of an algorithm, from the formulation of the problem to be addressed to the interpretation of its results. These examples, and many others, show that eschewing bias in algorithms would require freeing society as a whole of bias.
You also highlight problems caused by facial recognition.
If you train a machine-learning algorithm to perform facial recognition, the quality of its predictions will depend in part on its exposure to a sufficient number of images representing different people (with different phenotypes, for example).
In some databases, faces from racialised people are far less represented than Caucasian faces. This can lead to absurd situations with potentially far-reaching consequences. For example, during the COVID-19 pandemic, some European universities used facial recognition software to prevent students from cheating when taking exams remotely. Robin Aisha Pocornie lodged a complaint against algorithmic discrimination because the software used by her university had trouble recognising her face. She had to take her exams with a light shining on her face for the software to work.
In another area, studies have shown the persistence of a pay gap between men and women working for digital platforms, for example in the transport sector, despite the use of pay algorithms that are programmed to disregard gendered criteria. A recent study in the United States found a 7 per cent pay gap between women and men working for the Uber platform. This bias is attributable to several factors, in particular speed and the areas where drivers decide to work. These choices are gendered: on average, women drive more slowly than men, and they choose to go to areas perceived as quieter, where there is less demand and the price of a trip is lower on average.
Finally, we cannot rule out the possibility that some algorithms are deliberately biased.
How can we fight these abuses?
The task is complex because the sheer size of the data used by algorithms and the replicability of the decisions they generate amplify discrimination on a large scale. What’s more, the automation of decisions via algorithms, be it in the public or private sectors, often stems from a drive to reduce time spent processing data, which in turn may result from a goal to cut staff, costs and/or increase productivity.
However, if the designers and users of algorithms are to control the quality of these algorithms, they need to be trained and given the time to test and scrutinise the outputs, requiring significant investment in these areas. The same applies to regulators, legislators and lawyers, who need to understand how these systems work in order to put in place the right safeguards and regulations.
How can European law serve as a bulwark against these abuses?
European law provides some answers because it prohibits direct or indirect discrimination based on six criteria: sex and gender, race or ethnic origin, religion or beliefs, age, sexual orientation and disability.
Returning to the issue of the gender pay gap among platform-based workers, in principle, European law prohibits paying women workers less for the same work. And the new European directive on improving working conditions on digital platforms will facilitate the application of this ban.
Are there other options?
Another option under consideration would be to make more systematic use of, or even strengthen, existing provisions on the reversal of the burden of proof.
In principle in European law, if an individual presents facts from which it can be presumed that algorithmic discrimination has taken place, it is for the user to prove that the system is not discriminatory. If platforms and companies had to prove the absence of discrimination before using an algorithmic system, they would have a greater incentive to take preventive measures when designing and deploying algorithms.
Could the Digital Services Act (DSA) recently adopted by the European Parliament help advance these initiatives?
The DSA is a step forward in protecting internet users, especially vis-à-vis very large online platforms and search engines. The Act tackles profiling and its consequences. For example, the DSA bans the targeting of advertisements based on profiling that uses sensitive personal data such as sexual orientation and ethnic origin.
This is progress, but given the scale and variety of algorithmic discrimination, much remains to be done.