Home>Is Generative Artifical Intelligence Yet Another Threat to Democracy?
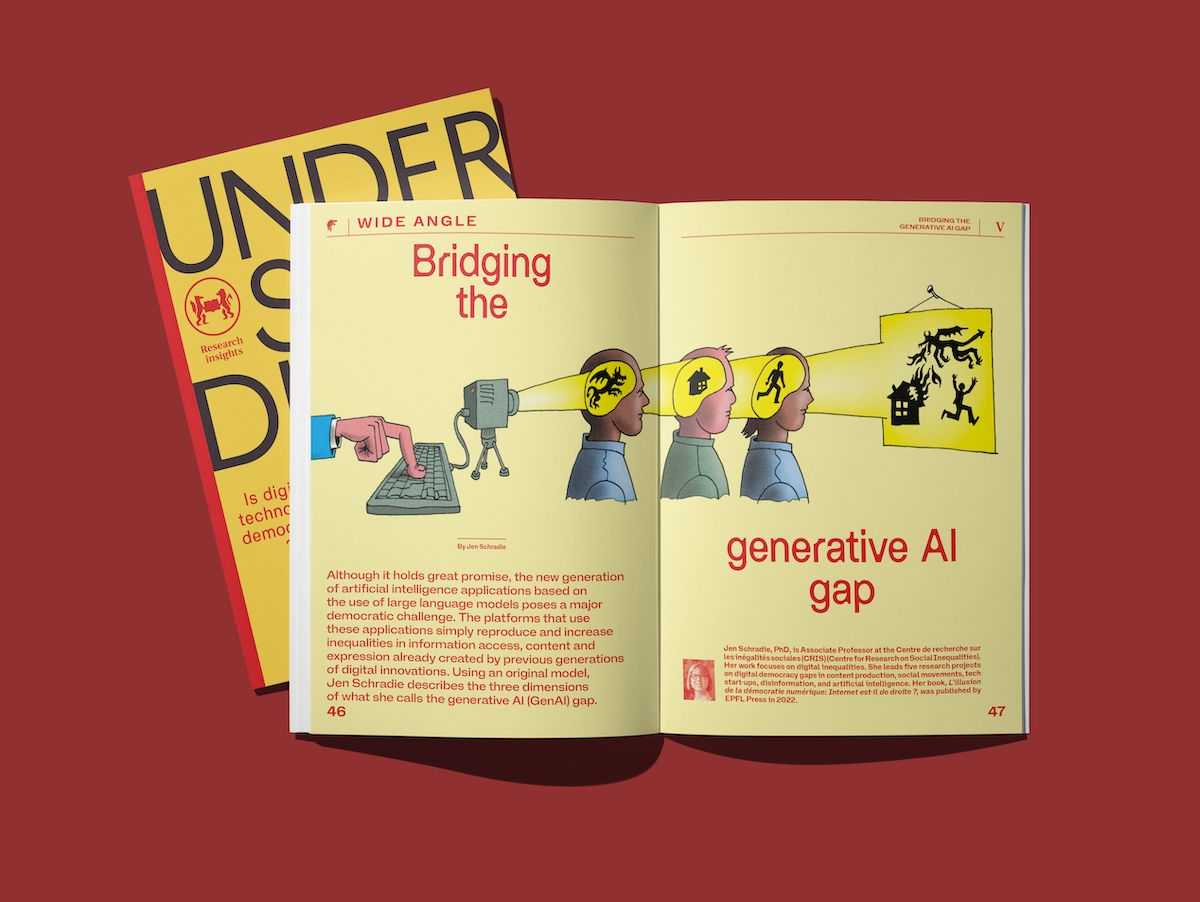
08.01.2025
Is Generative Artifical Intelligence Yet Another Threat to Democracy?
Although it holds great promise, the new generation of artificial intelligence applications based on the use of large language models poses a major democratic challenge. The platforms that use these applications simply reproduce and increase inequalities in information access, content, and expression, already created by previous generations of digital innovations.
Using an original model, Jen Schradie, PhD and Associate Professor at Sciences Po Centre for Research on Social Inequalities (CRIS), describes the three dimensions of what she calls the generative AI (GenAI) gap. This article was originally published in the second issue of Understanding Our Times, Sciences Po Magazine.
flip through the full magazine
Have you used an OpenAI platform such as ChatGPT? What about Apple’s Siri or Amazon’s Alexa? Ever interact with a chatbot? The integration of Artificial Intelligence (AI) platforms into our digitised society is not new. But AI is riding another wave of hype thanks to the mainstream availability of products built on Large Language Models (LLMs). Until recently, AI served primarily as an advanced data analysis tool. LLMs take this a step further by ingesting vast amounts of content that is used to “train” the AI, so it can generate new content or predictions based on plain text “prompts” from users.
This Generative AI, commonly called GenAI, has created the classic euphoria that often comes with new technologies. One of the most popular services built on LLMs, ChatGPT, became the fastest growing app in the history of the internet. GenAI partisans insist it can be used to do everything from writing blog posts to developing code to making movies to diagnosing patients to, well, the list is infinite.
Amid this rapture, GenAI has created a host of new problems that are starting to get more attention from the public and policymakers. Those LLMs are fantastically expensive to operate. The computing power required has caused an explosion in energy consumption that has led many leading tech companies such as Google and Microsoft to ditch their decarbonisation goals. And the wanton and often unauthorised use of other content online to train the LLMs models has raised troubling questions about privacy and creators’ rights that have triggered calls for more regulation and an avalanche of litigation.
Yet there is a key democratic challenge that GenAI presents that, until now, has not received sufficient scrutiny: GenAI platforms are replicating and magnifying social and class inequalities found in the real world. Previous cycles of technology – most recently the internet and smartphones – have initially been greeted with utopian hopes that they would make societies and economies more democratic, only to give rise to information inequalities. In the end, social class and ethno-racial differences in education, income, resources, and infrastructure form the basis of key disparities that determine who benefits the most from new information and communication technologies.
This is even more true for GenAI. Just as its adoption is happening at an unprecedented rate, the information inequalities being created by GenAI platforms are happening at a scale never seen before. I call this the GenAI Gap. As a researcher at Sciences Po, I study such information inequalities using my Modèle Lumières pour l’égalite numérique (Enlightenment Model for Digital Equality).
The framework that I have developed rests on three pillars: Participation, Perception, and Representation. Only by understanding the democratic challenges posed by GenAI will we be in a better position to tackle them.
GenAI and the Enlightenment
On a fundamental level, the knowledge gaps that define the digital era are the same that people have struggled to overcome in order to create more equitable societies for centuries.
French philosopher Denis Diderot understood the power of democratising information during the eighteenth century. In an effort to challenge the power of the ruling royal and theological elite, he strove to make knowledge more accessible by gathering and structuring information to reach a broader audience. With other writers, Diderot published the Encyclopédie, books that were not simply Biblical or literary but a fresh approach to factual data that contested the authorities, namely the church. But Diderot and his co-authors were not acting alone. This project was supported, in part, by les Lumières, the French Enlightenment philosophers, who directly influenced the groundswell of support for the French Revolution by building their own information communities.
As noble as these efforts were, three class-based information inequalities plagued non-elites in the eighteenth century. First, not everyone could participate in accessing and reading the philosophers’ content, due to limited literacy or funds to buy books. In addition, limited perception abilities constrained many in the 1700s in discerning fact or scientific information, rather than mythical or religious of nature. Finally, despite Diderot’s and his co-authors’ efforts, these books and writings lacked adequate representation of the lives of the poor, compared to those who were better off – not everyone’s stories were told.
While these information inequalities have diminished over the years, especially in France, they have nonetheless persisted. Now, in the GenAI era, these types of inequities are exploding, threatening the foundation of democratic societies. Using the Enlightenment Model for Digital Equality, let’s examine the core characteristics of the emerging GenAI Gap.
The participation gap
A primary factor in the GenAI Gap is that not everyone can participate with these platforms at the same pace. I found a similar phenomenon in my book, L’Illusion de la démocratie numérique, which analysed a digital activism gap – people from higher social classes and white activists, who were using the internet for social movements and politics, were more likely to have what I call ASET: Access to these digital tools, the Skills to use online platforms, feeling Empowered to post to the internet, and having the Time to use tech effectively.
I am applying these principles to a current comparative information project, with an eye toward GenAI. Based on preliminary survey data for research I am conducting in France and the United States, only about half of respondents use ChatGPT, for example. One could argue that it is simply a matter of time before new technologies will reach wider adoption. But new platforms and services are constantly being introduced. Early adopters, who tend to come from more elite classes, typically stay ahead of the digital curve.
Along with a research team I organised, we are using a combination of computational, quantitative, qualitative data to understand how these ASETs apply to GenAI:
- How do social class differences – people with more education and income – shape the way they access the firehose of platforms that emerge on the scene every day? The proliferation of GenAIpowered services can make it difficult for even the most resourceful professionals to keep up.
- How do skills play a role in the ability of people to harness the power of GenAI?
- How confident and empowered do people feel using GenAI?
- Finally, do people have the time to learn about these tools and hone the expertise to take advantage of this AI firepower?
The perception gap
“The first step towards philosophy is incredulity”, wrote Diderot, referring to the value of adopting a sceptical view of all dominant thought. Indeed, the second aspect of the GenAI Gap is the difference between those who have and do not have the educational background, training, and experience in developing a keener perception of what is factual information generated by GenAI platforms versus what is false, or more commonly called “hallucinations”.
In ChatGPT, for instance, if you ask for an answer to a question, and it cannot readily formulate an accurate response, it will make up an answer that is false. So extra work and skills that involve astutely questioning any GenAI-generated content is essential to using information in a more democratic, and less authoritarian, way.
In my current research on disinformation, this is a key question that we ask respondents, with only one-third of people in France and the U.S. showing concern about AI as a pathway toward disinformation. While certainly political ideology and other factors can shape perception, these class constraints are key.
The representation gap
Finally, the GenAI Gap is fundamentally widened by the inequality of voices that are used in the LLMs from which GenAI platforms draw – and those whose voices are missing. This is a question of representation. While many justifiably bemoan the massive theft of content that AI pillages, just as problematic the content that is not being included to train these models.
Over a decade ago, while digital divide scholars were focused on demographic differences in the consumption, or access, of online content, I showed empirically, as well as theoretically, the importance of looking at inequalities along the lines of who was producing online content and who was not. Simply, people with higher education levels are more likely to be represented online based on higher levels of digital production. Therefore, if LLMs draw from existing online content, we are already starting out with more elite voices online who will then dominate GenAI responses.
I found similar gaps in my research on digital activism – finding that social movement groups and activists who came from more privileged backgrounds produced more digital content. If we only look at elite voices in social media as to what is key or trending, we are missing marginalised voices. In the same vein, only viewing responses that are trained on incomplete LLMs limits people’s representation in the digital public sphere.
In other words, overlooked information is missing from the new corpus of text, image, sound and video data that AI is generating since the content it is consuming to “learn” from and then generate is based more on elite, rather than marginalised, voices. This GenAI representation gap is a direct threat to democracy and is the focus of my next GenAI inequality research project that will trace and evaluate these gaps in the content itself and its implications for society.
From the minitel to other public AI strategies to tackle the GenAI gaps
By understanding the mechanisms of the GenAI Gap around participation, perception, and representation, we will better be able to identify the solutions to overcome them – whether resource, educational, or other findings that need to build on previous research and historical lessons.
For example, when France launched the minitel, it did so with a strong public foundation that enabled people to access a French internet at low cost, unlike many countries, such as the United States. In the process, France took advantage of developing technology to democratise information. Continuing this public approach in tackling the new GenAI Gap will go a long way towards addressing these inequalities.
Yet new methods and collaborations are also essential. As Diderot said, "Il faut tout examiner, tout remuer, sans exception et sans ménagement." (We must examine and stir up everything, without exception and unsparingly.)