Home>Using Digital Traces to Enforce Platform Regulation
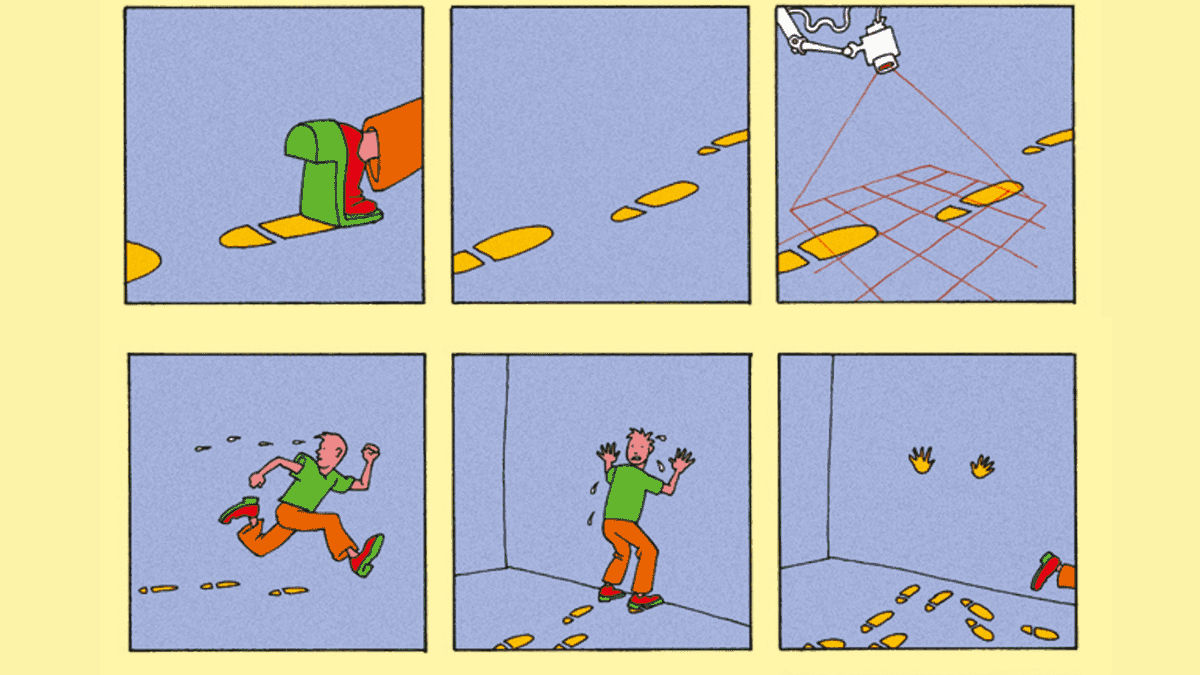
12.02.2025
Using Digital Traces to Enforce Platform Regulation
The geometric representation of the positioning of political parties and individuals according to different dimensions, a standard feature of comparative political studies, has only recently emerged in the analysis of digital data.
The visualisations presented here by Pedro Ramaciotti, Researcher at Sciences Po médialab and the Centre national de la recherche scientifique, Head of the European Polarisation Observatory, Jean-Philippe Cointet, Professor at the médialab and Director of Sciences Po Open Institute for Digital Transformations, and Tim Faverjon, PhD student at the médialab, are based on analyses carried out on the digital traces of X/Twitter accounts. This research opens up avenues for regulators to prevent the risk of political profiling of platform users without their knowledge.
This article was originally published in the second issue of Understanding Our Times, Sciences Po Magazine.
flip through the full magazine
The proliferation of exchanges via social networks and the democratisation of automatic learning algorithms, which ‘calculate’ individuals on the basis of their behavioural traces, are giving rise to growing mistrust.
These technologies, which define the form and rules of interaction within the digital public space, are accused of increasing the polarisation of debates, encouraging the proliferation of hate speech and spreading disinformation (fake news), among other issues. Such fears underscore the need to focus on existing regulatory mechanisms to guarantee democratic principles.
Since the mid-2010s, Europe has created an innovative regulatory framework through a series of legal instruments such as the Artificial Intelligence Act, the Digital Services Act (DSA), the Digital Markets Act (DMA) and the General Data Protection Regulation (GDPR). Two of these – the GDPR and the DSA – seek to protect European Union (EU) citizens from intrusive data collection and advertising that uses personal information such as ethnic origin, sexual preference, religion and political opinion (Article 26.3 of the DSA, which refers to the list of sensitive categories from Article 9.1 of the GDPR).
On 14 March 2024, less than a month after the DSA came into force, LinkedIn was censured by the European Commission, which suspected the platform of using sensitive data (including political preferences) from users to expose them to targeted advertising. Article 34 of the DSA also requires platform operators to assess the risk that their services, including recommendation and moderation systems, pose to ‘freedom of expression and information, including freedom and pluralism of the media’. Europe’s leading role in protecting democratic principles online is laudable.
It is nonetheless legitimate to question the effectiveness of these legal tools. The DSA prohibits platforms from engaging in political profiling for advertising purposes, but what tools does the regulator have to detect this type of profiling? Similarly, social networks are given real responsibility for the variety of opinions visible online. However, the amplification systems that make the algorithms so addictive are also likely to produce an incomplete or biased view of opinions.
So how to identify and quantify this deviation from the pluralist ideal? How to measure the diversity of opinions expressed on a given subject? The problem is twopronged. First, the information space to which users are exposed through the prism of the platforms needs to be observable. Second, the space in which respect for political diversity is desirable needs to be clarified. How should this diversity be measured? Should the ideological indicator be based on the right-left spectrum? Or should it be gauged in other attitudinal dimensions linked to sometimes emerging issues such as immigration, globalisation, cultural and environmental issues?
Measuring the opinions of large populations using their digital footprints
While it is common practice in comparative politics to use geometric representation to position parties or politicians along predefined axes, this type of practice has only recently emerged in the analysis of digital data. The nature of this data, generally resulting from behavioural traces left by individuals, depends on each platform; it typically includes information on what users share, write or ‘like’. They are of particular interest when they are produced by large populations of users, enabling conclusions to be drawn about national political systems on a large scale with greater robustness.
Using behavioural traces to estimate the positions of individuals according to ideological dimensions or spectrums (opposing right and left, for example) or positions (for or against) on various public policies is a relatively old practice. In the 1980s, pioneering work used parliamentary voting data to position legislators on ideological spectrums. The intuition was that legislators voting for the same laws were probably very close ideologically. Conversely, if their votes were rarely in agreement, then they were very far apart. Gradually, all these patterns of behaviour created a political space that enabled each player to be finely positioned in a one-, two- or even multi-dimensional space. The same is true today of digital traces, which can betray the political preferences of users when we collect the media they retweet or the accounts of politicians they follow (to mention only the case of X/Twitter).
The European Polarisation Observatory (EPO), led by Sciences Po, is tackling the measurement of the public opinion of large populations (from hundreds of thousands to several million users per country) based on their digital traces. While the first studies using social network traces, mainly sought to position individuals and content on spectrums opposing liberals and conservatives (particularly for political analysis in the United States), the research carried out within EPO seeks to extrapolate these studies for the different national contexts in the EU.
Statistical inference methods are developed using various databases that have been used to characterise the political space defined by the parties in each country. For example, data from the Chapel Hill Expert Survey are used to position the political parties on dozens of ideological dimensions or public policy issues that structure each national context: right-left, European Union, immigration, confidence in institutions and elites, etc. This expert data enables validation and calibration of the results obtained by analysing digital traces and, above all, expansion of this classification to the party level across very large populations.
Measuring online behaviour and exposure according to political preferences
Because their political positioning has been estimated along dimensions specific to their national contexts, and because these estimates are linked to digital traces (unlike, for example, traditional survey data), these populations could become a primary source of metrics for the regulator to assess political profiling. This is illustrated by two studies published in 2023 and 2024, respectively: one on the relationship between polarisation and disinformation online, and the other on algorithmic content recommendations on social media.
Online misinformation is one of the central issues in moderating and regulating platforms. Understanding the determinants of fake news sharing is key to fighting disinformation better. Research carried out in the United States has shown that disinformation is mainly spread by a small share of the population on the fringes of the political spectrum, and particularly on the far right. The populations produced by EPO at an EU level enable an extension of the results obtained in the United States to other countries, accounting for the specific political dimensions that structure their digital space.
The best illustration of these results is the aforementioned 2023 study, which analysed misinformation circulating on X/Twitter. It shows that in France fake news-sharing behaviour is largely determined by the position of accounts along two independent dimensions: on the one hand, the right-left axis, and on the other (and perhaps above all), the anti-elite sentiment and distrust of institutions harboured by certain accounts.
Analysis of algorithmic content recommendations further illustrates the challenge facing regulators. To comply with Article 34 of the DSA, platforms must assess the impact of algorithmic recommendations on plurality and freedom of access to information. In countries where X/ Twitter is the platform of choice for journalists and political figures – as is the case in almost all of Western Europe and on the other side of the Atlantic – it is easy to imagine the consequences of targeted algorithmic amplification that would favour or penalise messages and content emanating from a single party or reflecting the perspective of a single political camp.
To analyse these issues, researchers, who are explicitly given this role by article 40 of the DSA, need to have access to both the data on platform recommendations and a political characterisation of the content recommended and the users to whom it is offered. This is the purpose of the 2024 study on algorithmic recommendations, based on digital populations produced by EPO, in collaboration with the CNRS (the ‘Horus’ project). By jointly assessing the political positions of the authors and recipients of recommended messages, this study provides the first quantitative assessment of the political diversity of recommendations to which players in the French Twittersphere are exposed.
It clearly shows (see figure above) that recommendations obey a logic of ideological segregation: users from the left, centre and right are overexposed to messages from their respective political camps, though to a lesser extent for centrists. In other words, messages published by friends who share the same opinions are systematically amplified by the algorithm.
The only exception to this boost for ideological proximity is that the algorithm also amplifies messages from far-left-wing users among right-wing users, to the detriment of content published by moderates. It is also interesting to note that the reverse is not true, and that left-wing users appear to be underexposed to content from the right (in almost the same way as content from moderates).
Can artificial intelligence inadvertently generate political profiles?
The digital traces of platforms enable building unprecedented bridges between computer science and comparative politics. A question that must be considered is whether the artificial intelligence (AI) algorithms used to recommend content on platforms might inadvertently build political profiles of users in their deep layers.
AI technologies exploit massive quantities of data and produce complex statistical models to calculate, for example, predictions or information rankings (which feed into algorithmic recommendations). However, these models are not always comprehensible or explainable, which is why they are often referred to as black boxes. Hence the risk that recommendation algorithms may unwittingly internalise political user profiles in their calculations. If so, how can this phenomenon be detected, measured and, if necessary, protected against? These questions are justified for two reasons.
First, the creation of profiles within AI models would constitute a breach of Article 26 of the DSA and would, in practice, mean an unwanted shift in the responsibility of platforms, which are hiding behind the opacity of the models. Detecting these profiles in AI models could also prevent intentional but stealthy breaches of Article 26.
For example, if the operator of a platform is convinced that its AI model will provide relevant political advertising to its users (by anticipating what content will be shown to users of a particular political persuasion), without having to make this explicit in the design of its AI model, it will be able to offer targeted political advertising as a service while claiming that the users’ political profile remains unknown to the machine.
Second, efforts to moderate the negative phenomena caused by the political diversity of the content consumed (such as exacerbated polarisation) raise complex normativity issues: what degree of content diversity should be imposed on users? Who should measure it and who should impose it?
In addition to revealing the political profiles of users, it is conceivable that these models could be used to selectively delete information that might betray an individual’s political preferences. Is it possible to design recommendation systems that are blind to politics, that comply with legislation, but that remain relevant to the user? Developing the ability to map the political space suggested by digital traces is key to answering this question. And it is crucial in this respect that digital platform data be widely auditable by research.